22 min read
Mastering Industrial AI: Tips from Bryan DeBois for Business Leaders
Sebastian Schieke
:
May 31, 2024 1:30:00 PM
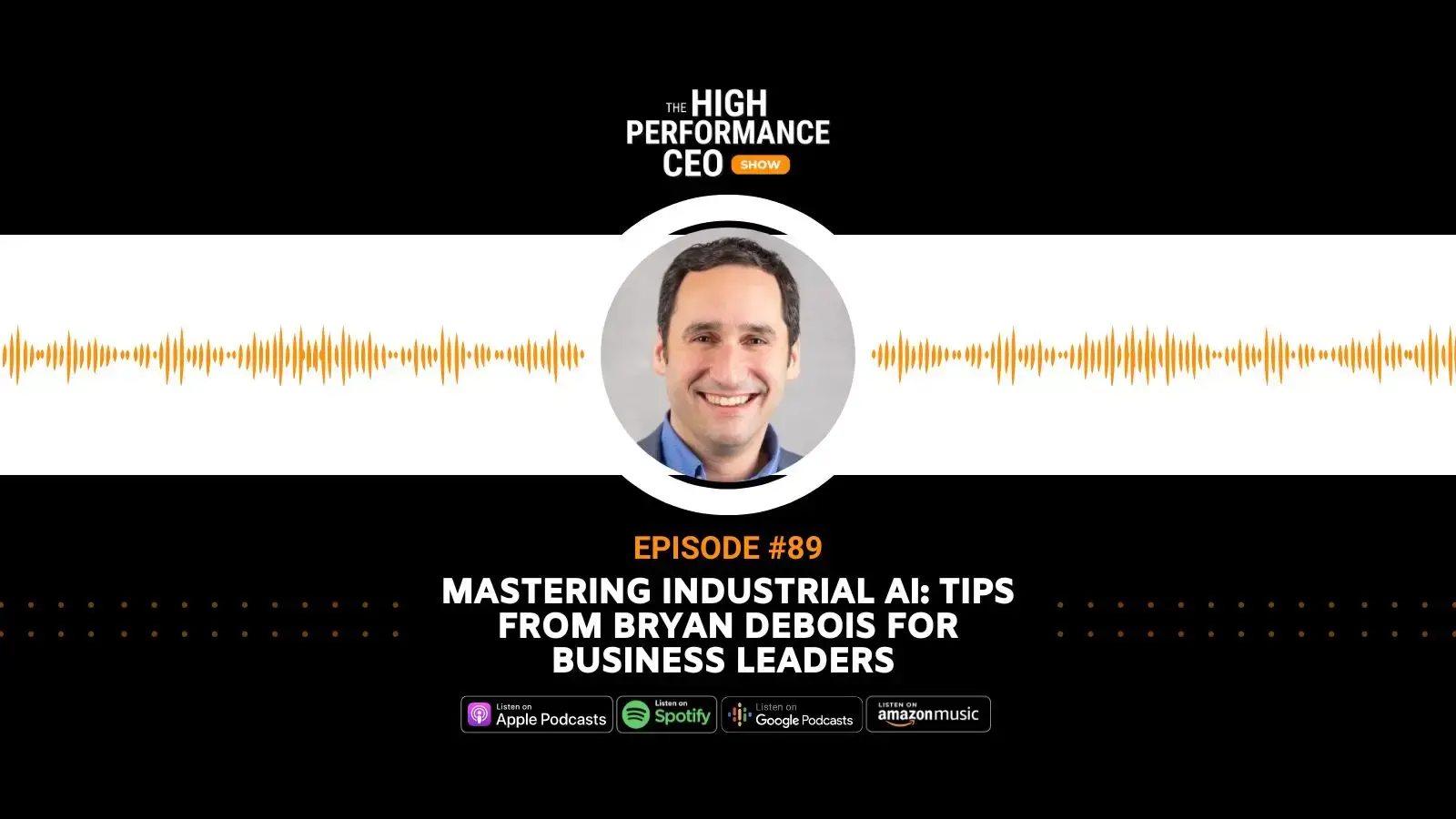
Episode Summary:
Industry expert Bryan DeBois shares practical tips on mastering industrial AI. This episode addresses the challenges and solutions of implementing AI, including managing stakeholders and deployment methods. Learn how deep reinforcement learning drives autonomous AI.
Essential viewing for CEOs and business leaders who want to excel in industrial automation.
About Byran Debois:
-
Extensive Experience in Industrial AI: Bryan DeBois has over ten years in the industrial sector, leading AI projects that optimized and automated complex processes.
-
Leadership Role at RovaSys: At RovaSys, Bryan has used deep reinforcement learning and advanced AI to solve real-world industrial problems.
-
Published Author and Speaker: Bryan shares his insights at conferences and has written several papers and articles on industrial AI, making him a thought leader.
Key Takeaways:
-
Understand AI's Impact: Learn how AI can automate and improve industrial processes to boost productivity.
-
Implement Smartly: Use effective strategies to integrate AI, including proper stakeholder management.
-
Stay Informed: Keep up with deep reinforcement learning and its effects on autonomous AI.
-
Learn from Experts: Use insights from leaders like Bryan DeBois to manage industrial AI complexities.
-
Lead Innovatively: Use AI to drive performance and innovation in your leadership, teams, and organizations.
Why It's Important for CEOs Like You
In this episode, Bryan DeBois talks about how AI can change the industrial sector. He explains complex AI ideas in simple, actionable terms. Bryan gives CEOs practical tips on using AI to streamline processes and boost productivity. The episode covers deep reinforcement learning and smart AI integration, showing the benefits of ongoing learning and expert knowledge. It's essential listening for leaders who want to use AI to achieve business success.
What CEOs Can Start Doing
-
Embrace artificial intelligence to stay competitive and enhance operational efficiency.
-
Implement smart AI solutions systematically to streamline business processes.
-
Foster continuous learning to stay updated with evolving AI technologies.
-
Utilize expert knowledge to navigate complex AI integration effectively.
-
Focus on leveraging AI to boost innovation and productivity within the organization.
Conclusion
This episode shows how AI is changing the industrial sector and why CEOs need to integrate it wisely. Key points include understanding AI's potential, using effective strategies, and learning from experts like Bryan DeBois. By embracing AI, promoting ongoing learning, and using expert knowledge, CEOs can foster innovation and boost productivity in their companies. This episode is a must-listen for leaders who want to stay competitive in a tech-driven world.
Chapters
Unlocking the Future: An Introduction to Industrial AI with Bryan DeBois 00:00-01:00
We introduce Bryan DeBois and discuss how AI is transforming industrial settings.
Meet the AI Pioneer: Bryan DeBois' Journey with Industrial AI 00:52-02:23
Bryan shares his background and his role at Rovasis in leading AI integration in industry.
Cutting Through the AI Hype: Real-World Industrial Applications 02:23-06:23
We talk about practical AI applications in industry, the challenges, and moving beyond the hype.
The New Era of AI: Introducing Autonomous Industrial AI 06:23-09:42
We explain autonomous AI, based on deep reinforcement learning, and its use in industry.
AI in Action: Step-by-Step Deployment in Industry 09:42-17:20
A detailed guide on implementing AI in industry, with stakeholder involvement and phased deployment.
The Future is Now: Next-Gen AI Solutions for Industry 17:20-28:32
Insights into future trends in industrial AI, covering operational and knowledge-based applications.
Reflections and Projections: Wrapping Up with AI Insights 28:32-End
Final thoughts on AI's impact in industries and future developments.
Read the full transcript here
-
Transcript
Sebastian Schieke (00:00.91)
Hey, Brian, welcome to the show.
Bryan DeBois (00:04.208)
Yeah, happy to be here.
Sebastian Schieke (00:05.614)
Yeah, it's an amazing time to live in and today we talk about AI again. I had so many interviews about AI recently. But it's really such an important and interesting topic. And I talked a lot about AI in the business world, in administration, for knowledge workers. But
Bryan DeBois (00:13.104)
I'll bet, yeah.
Sebastian Schieke (00:27.406)
Today is a very interesting focus in HCI for industrial appliances, for an industry, and you're an expert in that. And this is why I think many listeners should really focus on this episode, because I guess we will learn a lot about the current state of AI in the industry, but also what is on the roadmap, right?
Bryan DeBois (00:52.912)
Yeah, that's exactly right. So my background, I am the director of industrial AI for a company called Rovasis. And we are a global system integrator for industrial and manufacturing customers. My background, I have a computer science degree and I've been with Rovasis my entire professional career. So about 24 years now in this space. And then about five years ago, Rovasis has been around for 35 years, but about five years ago,
we saw an opportunity to start to approach these AI types of projects in the industrial space. And we really felt like what was happening was that there were a lot of AI vendors that were starting to come into this space and they were selling a certain story, dare I say hype to some of our clients. And we felt like there was an opportunity for us as a system integrator, independent system integrator to come in.
and really kind of set the record straight, as well as assist these AI vendors in actually deploying these projects in a running plant. So getting a model, it's one thing to design a working model, it's another thing to operationalize it into a running plant that needs to operate 24 seven and have all of the organizational change management that's required to get the buy into that. So.
That's kind of the background on that. And yeah, happy to talk about any and all aspects of that today.
Sebastian Schieke (02:23.95)
Yeah, I think you mentioned a very important topic. It's this hype or this perceived hype. I would say it's a perceived hype because I think it's more than a hype. It's such a revolution we're going through. But when I look in the last, yeah, I don't know, decade or so, we had so many hypes, so many, oh, this is the next big thing and everyone was focused on it and was going for it. And then we realized, okay, it was maybe a hype. And this is...
I think this is what is holding back people at the moment in really going full in on AI.
Bryan DeBois (03:01.168)
Yeah, and that's understandable. I think that, you know, one of the challenges is that most of the information that they get are from one of two sources. They're either from the AI vendors themselves. Well, of course, they've got all the motivation in the world to sell a certain story or from these analyst groups. And I have a lot of respect for the analyst groups. But the reality is, is that these analysts are not actually deploying these solutions. They're not on the plant floor actually trying to get this stuff to work. So you've got.
two different sources of information, and neither one of them really knows anything about how to actually get this stuff working in the real world. And so I do think that there's an important role for system integrators to come in and kind of sift through that. Now, that being said, despite the hype, we have a long track record now over five -plus years of implementing these.
AI solutions and advanced analytics solutions in the plant floor. So we can talk about that today and how we've got real stories where customers can see real value in doing this. So I do think that it's something that customers need to start taking the first steps on. But we are very much a walk before you run type of organization here. So I'd much rather see a customer just dip their toe into it and try out some things and
we have a very deliberate process for how we walk customers through getting there so that we de -risk at each step so that they can feel like, okay, I can buy into the next step and I can buy into the next step. You know, we're not one of those organizations that comes in and says, give us $12 million and we'll deliver AI. Like, that's not... Yeah, that's right. That's right.
Sebastian Schieke (04:46.51)
Yeah, you do it for 10. What is the typical use case in the industry for AI? I mean, what is the typical kind of client of you look like?
Bryan DeBois (04:59.664)
Well, let's talk about industrial AI as it's existed. So AI is not, despite what everyone kind of thinks, AI in the industrial world has existed and most frequently it's existed in the form of what's called predictive maintenance. So that's the one that everyone is pretty familiar with. Honestly, it's been around for 15, 20 years. And so that's basically where, you know, the goal is to be able to predict when this piece of equipment is going to go down.
Sebastian Schieke (05:02.926)
Mm -hmm.
Sebastian Schieke (05:15.726)
Mm -hmm. Yeah.
Bryan DeBois (05:29.392)
So be able to make a reasonable prediction that within 10 days or 15 days or 30 days, based on vibration and other characteristics, that this piece of equipment is going to go down. That's been around for a while. Predictive quality hasn't been around for quite as long, but that's also one that's pretty well established. And that's based on certain characteristics. I'm going to be able to build an ML model that will predict what the final quality of this batch that we're making is going to be. And if you can predict what the final quality is, what's the value in that? Well,
A lot of times you can start to do some rework or you can start to turn some knobs and change some inputs to get it back to on -spec product before it ever even gets fully produced. So those things have kind of been around for a long time. The one that we're focusing on, the type of AI that we're focusing on right now is called autonomous AI. And that is actually something new.
Sebastian Schieke (06:23.374)
Mm -hmm.
Bryan DeBois (06:26.672)
So a little background on that. So that is built on deep reinforcement learning, which if your listeners aren't familiar, deep reinforcement learning was a novel AI algorithm that came out of DeepMind, which was a Google spinoff. If you remember around 2016, 2017, there was all this hype around the...
Sebastian Schieke (06:40.142)
Okay.
Sebastian Schieke (06:45.166)
I don't know if I can pronounce his full name, but Mustafa. And he wrote this book, The Coming Wave, which is amazing. Pardon me?
Bryan DeBois (06:52.592)
About deep reinforcement learning? Was it about deep reinforcement learning?
Sebastian Schieke (06:57.198)
Nobody was. He's the founder of DeepMinds.
Bryan DeBois (06:59.824)
Oh, yes. OK, yes. And so that was really, and they've done some other stuff, but that was really kind of their big contribution to this space was this new algorithm. And they used it to build AlphaGo, which beat our best Go players. And they built AlphaZero, which beat our best chess players, if you remember. And so this was many years ago now. But there were some enterprising folks who said, well, we could take this thing and instead of applying it to solving, to playing games, we can.
Sebastian Schieke (07:02.83)
Yeah.
Sebastian Schieke (07:06.414)
Mm.
Sebastian Schieke (07:15.886)
Yeah.
Bryan DeBois (07:27.6)
take it and apply it to solving industrial problems. And so then that was kind of the thread. And then it went through Microsoft Research. And that was actually how Rovers got involved was through Microsoft Research. And then finally now it's come to fruition. And so we've got so deep reinforcement learning is part of autonomous AI. There's some other aspects to it that we can get into. But that really is what we consider the next generation of industrial AI. And it sounds futuristic. It seems like it can't.
possibly exist today. It seems like something that's still down the road, but we have actual customers that have it deployed and are solving production problems with this autonomous AI. What makes it unique is that it does what everyone wants AI to do, which is that it doesn't just make a prediction. It actually says, based on the current state of the system, here's your next best move. Here's your next most optimal move. And that's really what our customers are looking.
Sebastian Schieke (08:27.79)
Interesting. And what kind of customers, what kind of industries are applying these solutions?
Bryan DeBois (08:37.424)
So it's pretty widespread. So we've got customers. We've got food and beverage customers that are doing this. But then from there, we've got a glass bottle manufacturer that's using this thing. We've worked with paint manufacturers. We've worked with a company that makes baby formula. And so what we really get excited about with this autonomous AI approach is that we can use a single technology.
to solve a lot of different problems, which we love that. As a system integrator, we're looking for, it reminds me of, if you're familiar with, in our world, we have these time series databases called historians, and they've been around now for 20, 25 years. And it reminds me of that same arc that we had with historians in that it was a single technology time series database, but it could solve problems for all kinds of different industries and solve lots of different use cases.
in any one client. That's what we're seeing with this autonomous AI approach.
Sebastian Schieke (09:42.574)
What is a typical process to implement it in your organization? I mean, I could imagine, sorry, a lot of also challenges and questions when you talk to the staff. How do you handle that?
Bryan DeBois (09:48.56)
Yeah, so, yeah.
Bryan DeBois (10:02.16)
Yeah, well, let's dig into one use case in particular, and I'll kind of walk you through how we got from ideation all the way to an implemented AI solution. So we were working with a food company now, so they make sausages. And one of the things that we're really kind of pushing with this is let's start to tackle the unsolvable problems.
Sebastian Schieke (10:09.262)
Mm -hmm.
Bryan DeBois (10:28.72)
Let's start to talk, tell us about the problems that you've never actually been able to solve in the past. So you either throw, you know, you just throw labor at it, you throw humans at it, or you just live with the fact that, well, you know, so in that particular case of the sausage company, they were always over stuffing their sausages in their casing. So they were selling more, they were giving away product effectively, right? So, and why? Because they had issues with moisture content and they were really concerned that,
Sebastian Schieke (10:50.382)
Yeah.
Bryan DeBois (10:57.072)
As they cooked these sausages, it would lose moisture, as you would imagine. And because the moisture content was so variable and they really didn't have a good handle on it, they were just overstuffing the sausages because you couldn't sell an underweight product. So, you know, the only thing they could do... So, again, this was an unsolvable problem. They had tried a lot of different things over the years and it just was like, yeah, this is just what we have to do. And we said, well, let's tackle that. Let's look at how can we apply AI to...
get just the right amount of moisture before it goes into the ovens so that you can deliver a perfect on spec sausage at the end of it. And so the first, so we went into this meeting and we identified these different use cases and this was the one that we decided. So that's kind of the first step is we do like it, we call it an AI workshop and we get all the right stakeholders. And there was this brilliant food scientist in the room and she said, well, here's a problem that we've never been able to solve. And so that got on the list. And so we on the whiteboard, we're coming up with these different ideas.
We're kind of ranking them. We've got this process that we do to rank them. And then we identified that this was the one that popped to the top. So then we go off and we do what we call a exploratory data analysis. So that's where we actually, you know, at that point, all we have is a bullet point. So the exploratory data analysis is where we actually put the meat on the bone, right? So that's where we actually flush it out into a, you know, a full -fledged project. And so we look at all their data sources. We say, okay, is your data going to give us enough
to actually be able to solve this problem for you? Is it predictive enough? Can we build? One of the aspects of autonomous AI is that learns by doing, not by data. So we have to build some sort of a simulator. And we build what are called data -driven models. So we build a simulator off their data. We also typically incorporate formulas and equations and things like that. But ultimately, we have to build some kind of rudimentary simulator. It doesn't have to be the most high -tech, you know,
Sebastian Schieke (12:34.478)
Mm -hmm.
Okay.
Bryan DeBois (12:53.584)
you know, realistic simulator, it just has to be a rough simulation. And then we, so we did that process. And we came back to them and we said, yep, we've gone through the exploratory data analysis, we feel like we can accomplish this. So again, we're de -risking at each step. So then we go and we go off and we build the simulator. And so we use their data, we leverage their data, we leverage their expertise to build a data -driven model. And that model then, we go through a validation.
a sim validation step. At the end of that, everyone signs off. Do you all agree that this is a close enough representation of reality? And they all go, yep, that looks good. Okay, again, so very, very deliberate process. So now we can hook up the deep reinforcement learning to that simulator and we can start to have it learn. Okay. And in particular, we were simulating moisture ads.
Sebastian Schieke (13:48.622)
Mm -hmm.
Bryan DeBois (13:48.848)
So while they were having issues with moisture content, they also were adding moisture at various parts of the process. And we said, no, wait a minute. OK, how tightly are you controlling those moisture ads? Well, we just have a recipe. We don't change that often. We just dump water in. OK, so there's your dial. What about if we could deliver? And we call it a neural network trained with this approach is called a brain. We said, what if we can deliver a brain that monitors all of those moisture ads and makes sure?
Sebastian Schieke (14:10.062)
Mm -hmm.
Bryan DeBois (14:17.776)
that we add only just enough rather than too much. And then you have the issues with having to overstuff and things like that. So we then go through a process of what's called machine teaching. So this is another unique aspect to the autonomous AI methodology. So machine teaching is where we work with their best experts, so this food scientist in particular, and we capture all of the hierarchy of decisions, the workflow that their best experts make.
on, you know, in this case, adding moisture, right? So, or in the case of the glass bottle manufacturer, okay, how do you turn these dials to make sure that you make perfect glass bottles? And we work with their subject matter experts and we capture all that in this process called machine teaching. So we're not actually training a single monolithic deep reinforcement learning brain. We're actually training a network of agents that all work together to solve this problem. That, by the way, that machine teaching piece,
Sebastian Schieke (14:49.902)
Hmm.
Sebastian Schieke (15:09.166)
Mm hmm. Yeah.
Bryan DeBois (15:15.888)
is also how we get explainable AI. Because now we have this workflow. And so if you need to figure out why the brain made the decision it made, you actually have a workflow. You go, oh, I see. It thought we were in this state. That's why it made this decision. We really want it going down this path over here. So we make a tweak. And we can, you know, so we actually get explainable AI as part of this whole thing, which is pretty cool. So then we train the deep reinforcement learning brain, the autonomous AI brain. And then we go through a process of validating that.
Sebastian Schieke (15:27.758)
Aw.
Bryan DeBois (15:44.879)
Once everyone signs off, they, yep, this is making at least as good a decisions as our best operators. And in some cases, better decisions than our best operators. Now we're ready to put it into production. The last piece there, the deployment step, we always put in as a decision support system first. So it's going to go in and it's going to recommend changes to the operator. The controller will make those changes. A human being will make those changes themselves. We always run that way first for us, you know, for months.
Sebastian Schieke (16:13.358)
Yeah.
Bryan DeBois (16:15.088)
And then most of our customers do want to go then to what's called direct control. And so eventually then we will actually switch it to where the brain is making those control system changes. But as you can see, like our approach is very different than kind of what you probably have heard from some of these, particularly the IT vendors that have come into the, into our space, into what we call the OT or operational technology space. You know, they kind of want to just slam something in there, which will get completely rejected by the operators. I can tell you right now.
Sebastian Schieke (16:44.142)
Yeah.
Bryan DeBois (16:44.432)
So, you know, whereas ours is a very meticulous process over time that, you know, de -risks it, that handles the organizational change management, we involve those operators, those people who are going to be using the system on a day -to -day basis, they're involved from the beginning. So that, because we know how these projects go, like if you try to slam, if IT tries to slam something in there, those operators have a hundred ways to undermine that system. So we got to make sure that they're bought in from the beginning.
Sebastian Schieke (17:07.758)
Of course. Yeah.
Bryan DeBois (17:11.632)
and we go through this very deliberate process. Anyway, that was kind of a long story, but that's kind of a, that shows you kind of from soup to nuts, how we got to a deployed solution.
Sebastian Schieke (17:20.622)
But it's very interesting. I mean, when I talk about these agents, where I can really see which agent is making which decision, and then you can fine tune it. And I mean, we also built agent for automating processes in business functions. But it's the same technology, and it's the same principle behind it. You have agents communicating with each other. And yeah, I mean, you want to fine tune it. You want to test it until you can really.
say, OK, let's go do it on your own and run with it. But it's an amazing feature to scale operations. You don't have to scale your team in the same way you might want to scale your whole operation when you have phases of additional work which you have to deliver. And.
It's something which I see more and more, these AI agents taking certain activities. How do you see this developing? And you say, hey, what trends do you see in the industry, in this space?
Bryan DeBois (18:34.48)
Well, so I look at kind of the future of industrial AI and two broad buckets. One of them is solving operational problems. And like I said, I think we've got the tools today. Autonomous AI gives us the tools to solve operational problems. And like I said, in a lot of cases, problems that we've never been able to solve before. So we have those tools today and we're doing that today. The other broad bucket is solving the knowledge problems in our industrial world. That's not there yet.
Sebastian Schieke (19:01.102)
Hmm.
Bryan DeBois (19:03.248)
But that's where LLMs, chat GPT style, generative AI style tools, that's where those will play. And those, I'm interested and excited to see how those will solve those knowledge problems. So one of the classic examples would be, if you can imagine a manufacturing facility, they've got hundreds, thousands of pieces of equipment and you have a maintenance team whose job it is to keep that equipment running.
Sebastian Schieke (19:09.006)
Mm.
Bryan DeBois (19:30.704)
Right. And so they often oftentimes I mean nothing in manufacturing. It's all old. Like it's just the nature of our world. Like they're very risk averse. If they're not making product, they're not making money. So they don't want to take down their line for anything. So they don't do a lot of upgrades if they can avoid it. So oftentimes, you know, this equipment is 25, 30 years old. And so you've got folks who, you know, the maintenance manuals might may have been physical and they may were scanned to PDFs and they're in.
Sebastian Schieke (19:37.518)
Yeah.
Bryan DeBois (20:00.528)
you know, and they're buried somewhere and some drive. So what people are looking at naturally is, well, how can we take all that maintenance knowledge and how can we capture all that and build some sort of a chat GPT style thing that could act like our best maintenance manager? The problem that we have today is with hallucinations. So if, if your listeners aren't familiar, hallucinations are what happens when an LLM just makes stuff up. And it's a big challenge.
Sebastian Schieke (20:02.35)
Oh.
Sebastian Schieke (20:09.038)
Put into a nail. Yeah.
Hmm.
Sebastian Schieke (20:21.518)
Hmm.
Sebastian Schieke (20:29.006)
And it's 100 % convincing. This is the way it is.
Bryan DeBois (20:33.456)
Yes, 100 % convincing. And that's the real scary part. And so when it happens in chat GPT, it's kind of funny, you know, or it makes a bad marketing blurb or it makes a bad poem and it just makes something up and it's kind of funny. In the manufacturing world, you could get someone killed. I mean, that's the reality of it, right? So you could blow something up. So we can't have it ever hallucinate. So we've got to be able to solve this problem. And one of the...
Sebastian Schieke (20:44.43)
Yeah.
Bryan DeBois (21:00.336)
the key aspects that LLMs are just not good at doing today is saying, I don't know. So if you were to ask it, how do I fix this piece of equipment, even if it didn't have that knowledge, it's going to say, oh, I know how to fix that piece of equipment. You just torque this bolt here, and you just do this, and you tighten this thing up here, and you're good to go, and it'll just make stuff up. So there's some challenges there. Now, I've seen a few. There's a lot of people attacking this space. So.
Sebastian Schieke (21:18.542)
and you press those two buttons.
Bryan DeBois (21:29.296)
someone's gonna come up with it. I've only seen really a couple vendors that I felt like actually had a realistic approach for how they could solve this problem, but it's gonna be a challenging problem. But again, that's kind of, I don't see that as an immediate thing. That's a year or two down the road, that's solving the knowledge problems. But what I'm saying to customers is, I know this sounds like sci -fi, but we can do these, we can solve these operational problems today and build you a brain that can act like your best operators.
Sebastian Schieke (21:42.286)
Yeah, it's...
Sebastian Schieke (21:58.478)
Yeah. And I mean, coming back to this knowledge problem, I think you can use it as an advisor, you know, where you say, okay, I have a bunch of documents, I put them in LLM, I ask my questions, and then I get answers, and then you get a reference to the document. And then in the end, you can go to the document and say, okay, you read it and you get the 100 % answer, but it's still better than going through countless of folders and looking for the document where...
this procedure is placed on.
Bryan DeBois (22:29.52)
Yeah, yeah. So the vendors that I feel are could realistically solve it. The one vendor I was talking to, they were like, we have a no hallucination policy, which I loved to hear. They led with that. Like, we understand that the the risk of hallucinations. And so basically, they said everything that the that the LLM says will have a reference back to you like it has to have a reference back to here's the page, here's the diagram.
Sebastian Schieke (22:53.934)
Exactly.
Bryan DeBois (22:59.12)
and so that you can go verify it for yourself. To your point, I think that that's the right way to approach a problem like this.
Sebastian Schieke (23:07.502)
And still, I think it will make a big difference to the way people work at the moment most of the times. It's pretty interesting. I mean, I see there's a lot of opportunity. I mean, we called it, coming back to the maintenance prediction, which you mentioned beginning in Germany, it's called Industry 4 .0, where we had this and predicting maintenance and especially for appliances which are far away.
Bryan DeBois (23:13.104)
Mm -hmm.
Bryan DeBois (23:27.728)
Mm -hmm.
Sebastian Schieke (23:37.614)
in a remote place. It's a very, very useful feature. But yeah, it goes much, much further and is interesting, interesting use cases for companies. I mean, it sounds like a bit like science fiction and you say, yeah, you know, give us a problem you never solved before.
Bryan DeBois (23:39.504)
Mm -hmm.
Bryan DeBois (24:00.72)
Right. Right. Yeah.
Sebastian Schieke (24:04.526)
But it's definitely a cool place. Maybe name us a couple of more examples of projects you worked on.
Bryan DeBois (24:12.848)
Sure, sure. So I was just at a plastic extrusion plant last week, and we did the whole AI workshop. And one of the really interesting, it's the same thing. We said, what are the problems that you've never been able to solve before? And so one of the things that they brought up is that, so if you can imagine extruding, and they're extruding, it's only several microns thick. So very, very thin plastic, right? Now,
despite being that it's very thin, despite being that thin, there is a, so there's a window where your plastic is on spec, right? So it has to be, it can't be too thin, it can't be too thick, it's in between this window. Now, their best human operators can keep it, you know, let's say more or less right in the middle of that line between, so it's, they're creating on spec plastic, great. What the brain can do,
is not just keep it on spec, just not in the middle of that, but it can actually go as close to the bottom of spec as possible. Now, why is that valuable? Because if you're in the middle of that spec or if you're even on the top end of that spec, you're giving away plastic, right? Thinner plastic would work just fine. It's still on spec. It's not going to break. It's still within the bounds of... So they're still delivering good product. But in the meantime, they're delivering more plastic than they need to.
Sebastian Schieke (25:33.166)
Hmm.
Bryan DeBois (25:37.872)
They're so it's called and I was at a vinyl extruding plant and they had the exact same problem. That's what's interesting about this is you see these same problems kind of come up and they call it heavy vinyl. It's on spec, but they're just giving away product. They don't need to do that. And so they're but the tolerances are so tight that that's not something a human being could ever like dial in. You're talking about not just moving it between two and one, but you're trying to go to two point, you know, one point seven, you know, that kind of thing on the dial. It's just not something.
And so it's just not even a thing that they really try to solve. Like it's just an unsolvable problem for them and they just live with the fact that they give away product. I'm like, I can build a brain that can bring those tolerances down and actually deliver on spec product and save you guys plastic. And that's worth millions of dollars every year. So that's one example, but we also can use the same thing, the same, these same autonomous AI brains to solve scheduling problems, completely different problem, same technology. So we did a project for a paint manufacturer.
Sebastian Schieke (26:33.646)
Hmm.
Bryan DeBois (26:37.616)
where they needed to, they had two human schedulers that were building their production schedule. Here's the orders we need to fulfill, how's the best way for us to fulfill those orders. So that's a production schedule, right? And they put that together and they would put together a schedule every day and it would take them about an hour. And this plant ran 24 seven. So if a piece of equipment went down, their options were either try to call one of those two human schedulers in the middle of the night and wake them up, or they would just run suboptimally. They just.
until one of them came in in the morning and could build them a better production schedule. We were actually able to train a brain. We built a simulation of their process with all built in all the randomness of, well, if you run it one time, it'll take this long. If you run it the next time, it'll take a little longer, a little shorter. We built in all that randomness from their historical data. And then we were able to build a brain that learned, we sat down with their expert schedulers through that machine teaching, taught the brain, here's the best practices.
and then let it loose and it was able to build production schedules as well as their expert schedulers. And you know, their schedulers, they go on vacation, they get sick, you know, they take breaks, they take lunch, just like this brain doesn't do any of that. And it was able to build a schedule in about a couple of minutes versus an hour for a human scheduler. So that's what I love about this. We can throw this same technology at a lot of different types of problems.
Sebastian Schieke (27:44.43)
Hmm.
Sebastian Schieke (27:58.03)
Yeah, I like this phrase, we build a brain. Prine the brain builder. Hey, thank you. Thank you so much. It's a very, I mean, interesting use cases. I learned a lot and I think there will be lots of happening in the next coming months and years. And yeah, I'd love to have you again on the podcast, maybe in a year's time and see how things have developed.
Bryan DeBois (28:03.056)
Yeah, the brain.
Bryan DeBois (28:27.312)
I'd love to do that too.
Sebastian Schieke (28:28.174)
Yeah. Thanks a lot and enjoy building brains.
Bryan DeBois (28:32.624)
I will do. Appreciate it. My pleasure. Thank you.